The Power of Predictive Analytics in Customer Service
In today’s fast digital world, businesses want to boost customer service and improve user experience. AI-driven predictive analytics helps businesses foresee customer needs. This tool helps companies give personalised recommendations. Often, these suggestions come before customers realise they need help.
AI is transforming customer interactions for companies. Predictive analytics is key to engaging customers early, raising satisfaction, and driving sales. This article looks at how predictive analytics affects customer service. It covers the benefits, strategies for implementation, and ways businesses can use AI insights to stay competitive.
Understanding Predictive Analytics in AI-Driven Customer Service
Predictive analytics uses past data, machine learning, and statistics to predict future customer behaviour, preferences, and needs. AI can spot patterns in customer data. This helps predict issues, suggest products, and make interactions smoother.
How Predictive Analytics Works
- Data Collection: AI collects vast amounts of data from multiple touchpoints. These include browsing history, purchase behaviour, customer feedback, and social media interactions.
- Data Processing: Machine learning algorithms analyse data to find patterns and trends.
- Predictive Modeling: AI uses stats to guess what customers might do next. This includes their favourite products or possible service problems.
- Actionable Insights: Businesses use these insights to personalise recommendations. They also optimise marketing efforts and improve customer service strategies.
Key Technologies Behind Predictive Analytics
- Machine Learning (ML): AI gets better at predicting things. It learns from past interactions to improve over time.
- Natural Language Processing (NLP): Helps AI analyse customer sentiments, feedback, and conversations.
- Big Data Analytics: It processes large amounts of both structured and unstructured customer data. This helps to find valuable insights.
- AI-Powered Automation: Uses predictive insights to automate responses, recommendations, and customer engagement strategies.
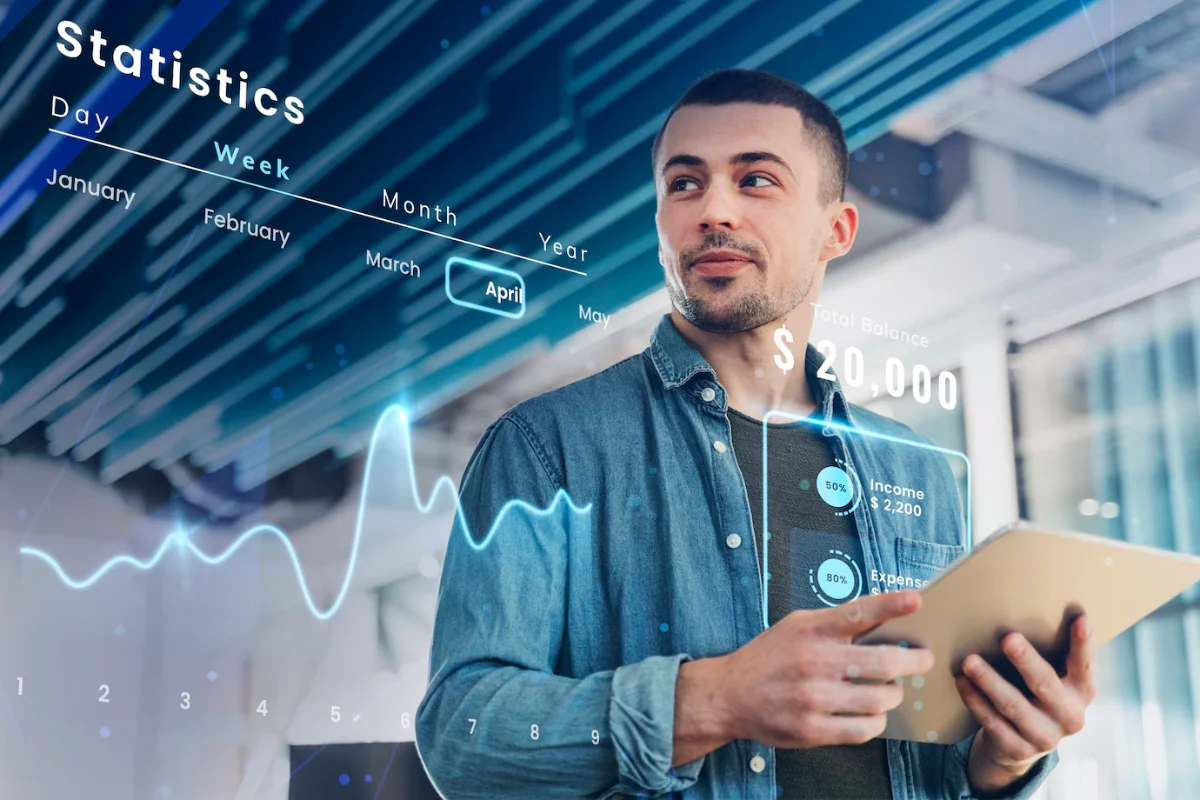
The Benefits of Predictive Analytics in Customer Service
1. Enhanced Customer Experience Through Personalisation
Predictive analytics helps businesses customise their interactions. It considers each customer’s unique likes and behaviours.
How It Works:
- AI analyses past purchases and browsing history to offer personalised recommendations.
- Virtual assistants use predictive insights. They anticipate customer questions and provide solutions before they’re asked.
- Chatbots suggest products or services based on a user’s real-time activity on a website.
Example: E-commerce sites like Amazon and ASOS use predictive analytics. They recommend products based on what customers have bought before. This boosts conversion rates a lot.
2. Proactive Problem Resolution
Predictive analytics helps businesses fix problems before customers even notice them.
Benefits:
- Identifies potential service disruptions and alerts support teams in advance.
- Detects signs of customer dissatisfaction and prompts businesses to offer targeted solutions.
- Reduces customer frustration by addressing pain points before they escalate.
Example: Telecom companies use predictive analytics to detect potential network issues. They notify customers before they experience connectivity problems.
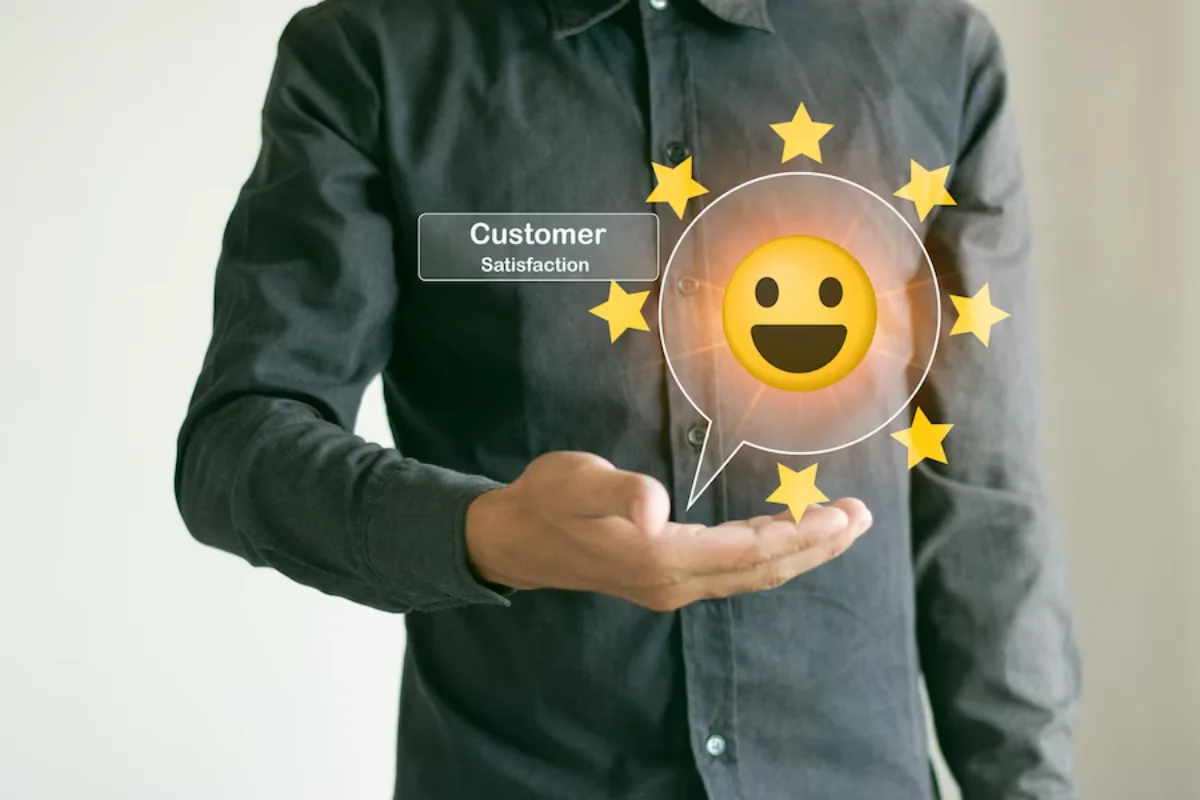
3. Improved Customer Retention and Loyalty
Businesses can boost loyalty and retention by understanding customer behaviour and preferences.
Strategies:
- Predictive analytics identifies customers at risk of churning and suggests targeted retention offers.
- AI-powered customer insights help companies create loyalty programmes tailored to individual shopping habits.
- Personalised email campaigns and exclusive discounts help keep customers engaged.
Example: Streaming services, such as Netflix and Spotify, study how users watch and listen. They suggest content based on these habits to keep users engaged.
4. Optimised Customer Support Operations
AI-driven predictive analytics enhances the efficiency of customer service teams by:
- Reducing response times through automated issue detection.
- Helping customer support agents prioritise urgent cases.
- Providing AI-powered chatbots with context-aware responses.
Banking institutions use predictive analytics to spot fraud. This helps alert customers right away, so they can avoid losing money.
5. Increased Sales and Revenue Growth
Predictive analytics boosts sales by identifying high-value customers and optimising marketing efforts.
Key Applications:
- AI suggests cross-selling and upselling opportunities based on customer purchase history.
- Predictive models forecast seasonal trends. This allows businesses to stock the right products in advance.
- Personalised promotions boost conversion rates. They do this by reaching the right audience at the right time.
Example: Retailers such as Zara use predictive analytics. This helps them find fashion trends that will boost sales in future seasons.
Implementing Predictive Analytics in Customer Service
1. Integrating AI-Powered Chatbots and Virtual Assistants
AI chatbots and virtual assistants use predictive analytics to improve customer interactions in real time.
Best Practices:
- Implement NLP-driven chatbots that understand customer intent and provide relevant solutions.
- Use AI to analyse chatbot interactions and refine responses based on common customer concerns.
- Ensure chatbots can seamlessly escalate complex issues to human agents.
2. Leveraging AI for Sentiment Analysis
Sentiment analysis allows businesses to gauge customer emotions and respond proactively.
Implementation Strategies:
- Monitor social media mentions and customer reviews to detect dissatisfaction.
- Use AI-driven feedback analysis to improve service quality.
- Identify common pain points and address them before they lead to customer churn.
3. Creating a Unified Customer Data Platform
To boost the power of predictive analytics, businesses should gather customer data from different sources.
Steps to Success:
- Integrate data from CRM systems, social media, and purchase histories.
- Use AI-powered dashboards to visualise customer behaviour patterns.
- Ensure compliance with GDPR and data privacy regulations to build customer trust.
4. Personalising Marketing Campaigns with Predictive Analytics
AI-driven insights can enhance marketing efforts by predicting customer responses.
Personalisation Tactics:
- Use dynamic email marketing campaigns based on customer interests.
- Recommend products through targeted advertisements.
- Optimise discount strategies by analysing past purchasing patterns.
Example: Fashion brands such as Nike and Adidas use predictive analytics. They send personalised product recommendations based on what customers do and like in fitness.
The Future of Predictive Analytics in Customer Service
1. Advancements in AI-Powered Predictive Models
Future developments in AI will enable more sophisticated predictive models, allowing businesses to:
- Forecast customer lifetime value more accurately.
- Identify emerging consumer trends before they become mainstream.
- Enhance chatbot intelligence with conversational AI advancements.
2. The Role of AI in Hyper-Personalisation
Hyper-personalisation will elevate predictive analytics. It will provide real-time, one-on-one marketing experiences.
Expected Trends:
- AI-driven voice assistants provide tailored recommendations.
- Augmented reality (AR) shopping experiences powered by predictive insights.
- Predictive pricing models adjust prices dynamically based on demand.
3. Ethical Considerations and Data Privacy
Predictive analytics needs a lot of data. So, businesses should focus on ethical AI practices.
Key Considerations:
- Transparent data usage policies to build customer trust.
- Compliance with global data protection laws like GDPR.
- AI models designed to avoid bias and discrimination in customer insights.
The Future of AI-Driven Predictive Analytics in Customer Service
Predictive analytics is changing customer service. It helps businesses anticipate needs, personalise experiences, and optimise support operations. Companies that embrace AI-driven customer insights will gain a competitive edge by offering:
- Personalised recommendations tailored to customer preferences.
- Proactive issue resolution that prevents service disruptions.
- Enhanced customer retention through targeted engagement strategies.
- Increased sales and revenue growth with AI-powered marketing.
AI technology is growing fast. Predictive analytics will soon be key in customer service. It will shape the future of personalised and smooth interactions.